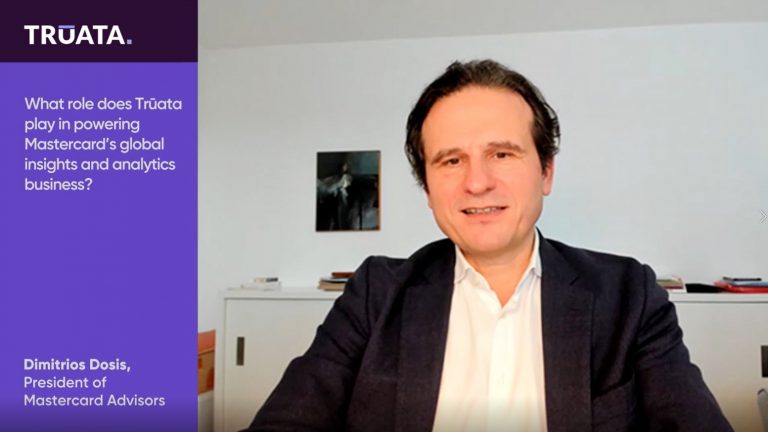
Mastercard & Trūata: shaping a data-driven future with a privacy-first mindset
President of Mastercard Advisors, Dimitrios Dosis, discusses the importance of powering data-driven innovation with a privacy-first mindset and how its partnership with Trūata makes this possible.