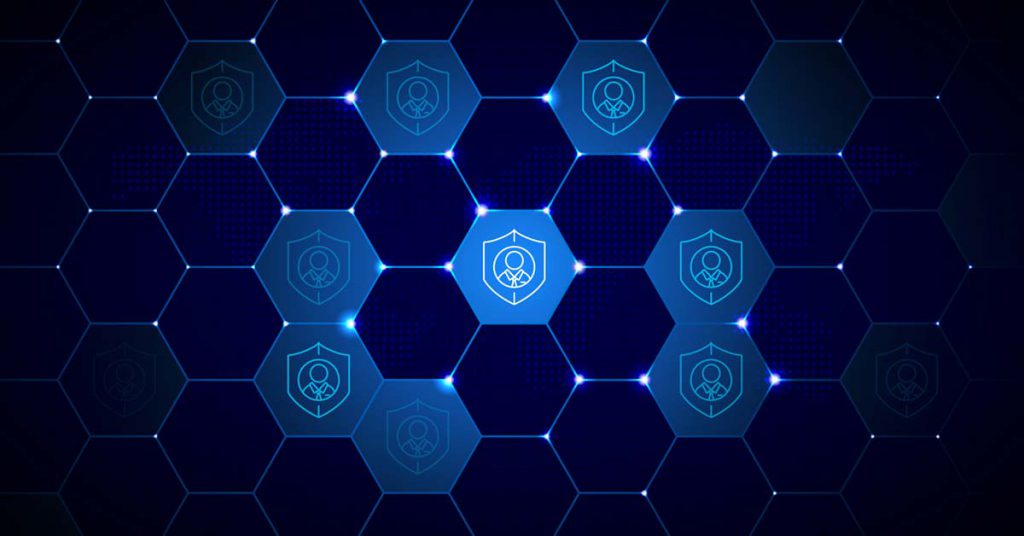
In our Big Data world, a world where artificial intelligence and machine learning “feed” off the ever-growing abundance of personal data, issues surrounding privacy and data protection are more prevalent than ever. However, while developments in technology are often seen as the crux of the problem, they can also be used as the solution, as evidenced in the recent international data transfer supplementary measures laid out by the EDPB. Pseudonymization is one such approach to overcoming the conflict that exists between the commercial need to utilize personal data and the need to protect the right to data protection and privacy.
The right to privacy: a snapshot
In response to recent technological leaps, the authors of a Harvard Law Review article, entitled The Right to Privacy, argue that the law needs to catch up with cultural, economic and technological changes. They note that companies “have invaded the sacred precincts of private and domestic life; and numerous mechanical devices threaten to make good the prediction that ‘what is whispered in the closet shall be proclaimed from the house-tops”. Interestingly, while the reference could apply to any of today’s tech giants, it actually dates back to 1890, just two years after Eastman Kodak revolutionized photography with the launch of the handheld camera – making it possible for people to take a photo of anyone in a public place at any time. This seminal article introduced the concept of privacy as a basic right and, not only did its title become the bedrock of many privacy opinions and regulations, it also triggered the interconnected relationship between privacy and technology that continues to permeate society today.
To tackle the issues that now exist in our tech-savvy but privacy-conscious world, great strides have been made to develop privacy-enhancing technologies (PETs) that allow the ferocious pace of innovation to continue while also protecting the consumer right to privacy and data protection. Under the GDPR and in non-EEA jurisdictions, one such approach to data protection is “pseudonymization”, which is used to achieve these dual goals. However, while the benefits of pseudonymization are being increasingly promoted by businesses and media, and it is indeed a powerful approach in most cases, it is also important to get to grips with some of its lesser-known characteristics in order to ensure that it is deployed effectively.
What is pseudonymization?
Pseudonymization is an approach to protecting the privacy of individuals in a given dataset by seeking to make it extremely difficult, or impossible, to re-identify an individual from a dataset without the use of additional information. This is achieved by transforming or processing either the entire dataset or individual columns to ensure that the resulting dataset is qualitatively different than the original. However, it is important to note that pseudonymization is not one technique or transformation, but rather a process that can be implemented in many different ways.
Depending on the risk profile and the desired analytical use, each field or record in a dataset can be distanced from its original value using a variety of data processing techniques that obscure or change the original value of a field. In using such data-processing techniques for the purpose of pseudonymization, re-identification risks can be removed. However, it’s important to know that pseudonymization has its limitations.
What’s the catch with pseudonymized data?
Pseudonymization can certainly be a powerful way to protect the privacy of individuals in a dataset, while still enabling analytics to be performed on the data. However, as with all privacy-enhancing technologies (PETs), pseudonymization must be approached with care and an understanding of what the underlying data processing techniques are doing. More importantly, the effect such techniques have on the re-identification risks of the data needs to be clearly understood.
Reducing re-identification risk can also impact analytical usefulness
Pseudonymization is not a silver bullet. As with all PETs, the degree of privacy protection added to a dataset affects the analytical utility of the resulting dataset; there is a correlative relationship between the two since obscuring the true value of a field reduces the ways in which it can be used for analytics and also reduces the precision of the analytical outputs. However, PETs have now evolved to such a sophistication that analytical utility can be preserved even when reducing re-identifiability.
When it comes to the techniques employed during the process of pseudonymizing data, it’s also important to be acutely aware of the impact of each technique. For example, perturbation is one such technique that alters a value using mathematical functions. However, perturbing a numerical field has an effect on the accuracy of analysis. As such, the best approach to selecting which transformations to apply is to have a deep understanding of which fields within a dataset contain the most re-identification risk so that targeted transformations may be made to minimize the impact on accuracy while also addressing the most likely risks.
This means that a data controller can make well-informed decisions around the use of their pseudonymized data based on a deeper understanding of the privacy risk and the impact on analytical utility.
The privacy-utility trade-off. Applying any form of pseudonymization impacts both privacy and utility characteristics.

One size does not fit all
Pseudonymization policies can be designed to preserve utility for specific use cases; however, preserving general analytical accuracy is significantly more challenging, if not impossible, for large datasets.
In fact, the solution for deploying pseudonymization within an organisation requires multiple pseudonymized versions of a source dataset to be created, with the types and degree of transformations tuned for each specific use case.
Pseudonymization at scale is challenging
In today’s data-driven world, data files can contain 50-100 columns as standard, and datasets containing many billions or trillions of records is considered the norm. This makes it not only impractical but almost impossible for a human to review and assess the re-identification risks contained in a data set.
In addition to this, relying on human intuition or expertise alone when deciding which transformations to apply as part of a pseudonymization will most likely lead to either unacceptable levels of residual privacy risk or the destruction of analytical usefulness of the data. Relying on a human to make effective decisions about which fields to transform and to what degree is near impossible without an objective, quantifiable risk assessment of the data set. To put it in perspective, in a data set containing 50 columns, the number of combinations of columns exceeds the number of stars in the Milky Way galaxy. This is why many organizations are turning to sophisticated tools to objectively quantify risk so that they can decide on targeted pseudonymization techniques for their large data sets.
Pseudonymization can prove to be ineffective in some cases
As already mentioned, pseudonymization policies can be designed to reduce the re-identification risk in a dataset while preserving enough analytic accuracy to deliver the desired business outcome. However, in some cases, the degree of transformation required is too great, especially if the riskiest fields in the dataset are those that require precise values for the use case in question. Due to the privacy-utility trade-off, it is impossible to completely mitigate all potential re-identification attacks, which means a risk-based decision must be made about the use of the data.
In instances where this happens, pseudonymization processes can be enhanced by limiting physical access at the level of the analytics interface. This implements the privacy-by-design principle through the separation of the analyst from the data. Such an interface can support familiar analytics techniques and workflows, yet prevent row-level data from being accessed.
Real-world application of pseudonymization
To enable high-value data analytics in a privacy-conscious way, pseudonymization requires careful consideration and precise deployment. Each use case or context will lend itself to a particular set of techniques that balance privacy and utility adequately.
One such example would be that of using pseudonymization for international data transfers, a topic very much of interest after the Court of Justice of the European Union (CJEU) ruled that data transferred to non-EEA territories must have the same level of data protection as if it were located in the EEA. Pseudonymization has been accepted and recommended as a supplementary measure to achieve the required level of protection for transferred data, so the application of pseudonymization techniques can be applied to support different types of transfer.
Ultimately, pseudonymization is an approach to privacy-enhanced analytics that seeks to obscure the true values of data fields so that they can be analyzed to drive business growth, while protecting individual data subjects from re-identification.
Want to learn more about how pseudonymization can be used as a solution for international data transfers? Reach out to speak with us directly if you are seeking automated solutions to improve efficiency, ensure compliancy, and drive business growth using your data.